Google Research and DeepMind Unveil Tx-LLM: A New Language Model for Therapeutic Development
Google Research and DeepMind have introduced Tx-LLM, a language model designed to predict the properties of biological entities throughout the drug discovery process. Tx-LLM, which was developed based on the PaLM-2 architecture, aims to support therapeutic research by improving the speed and accuracy of property prediction for entities such as small molecules, proteins, and cell lines.
Background and Purpose
The traditional drug development process is known to be time-consuming and costly:
- Typical development timelines: 10–15 years.
- Estimated costs: $1–2 billion.
- High failure rates in clinical trials.
These challenges arise from the complexity of ensuring that therapeutic candidates meet various criteria, including specificity, efficacy, safety, and scalability. Measuring these properties experimentally is resource-intensive, creating a need for alternative approaches.
Key Features of Tx-LLM
Tx-LLM was designed to address these challenges by leveraging machine learning (ML) techniques to predict therapeutic properties more efficiently. The model offers several unique features:
- Data Utilization: Trained on 66 datasets spanning the entire drug development pipeline, from early-stage target discovery to late-stage clinical trial approval.
- Comprehensive Functionality: Capable of handling a wide range of tasks, including:
- Classification (e.g., predicting toxicity).
- Regression (e.g., estimating binding affinity).
- Generation (e.g., predicting chemical reaction outcomes).
- Performance: Achieved competitive performance on 43 out of 66 tasks and outperformed existing models on 22 tasks.
- Versatility: Demonstrates strong capabilities in combining molecular data with textual context, enabling cross-domain predictions.
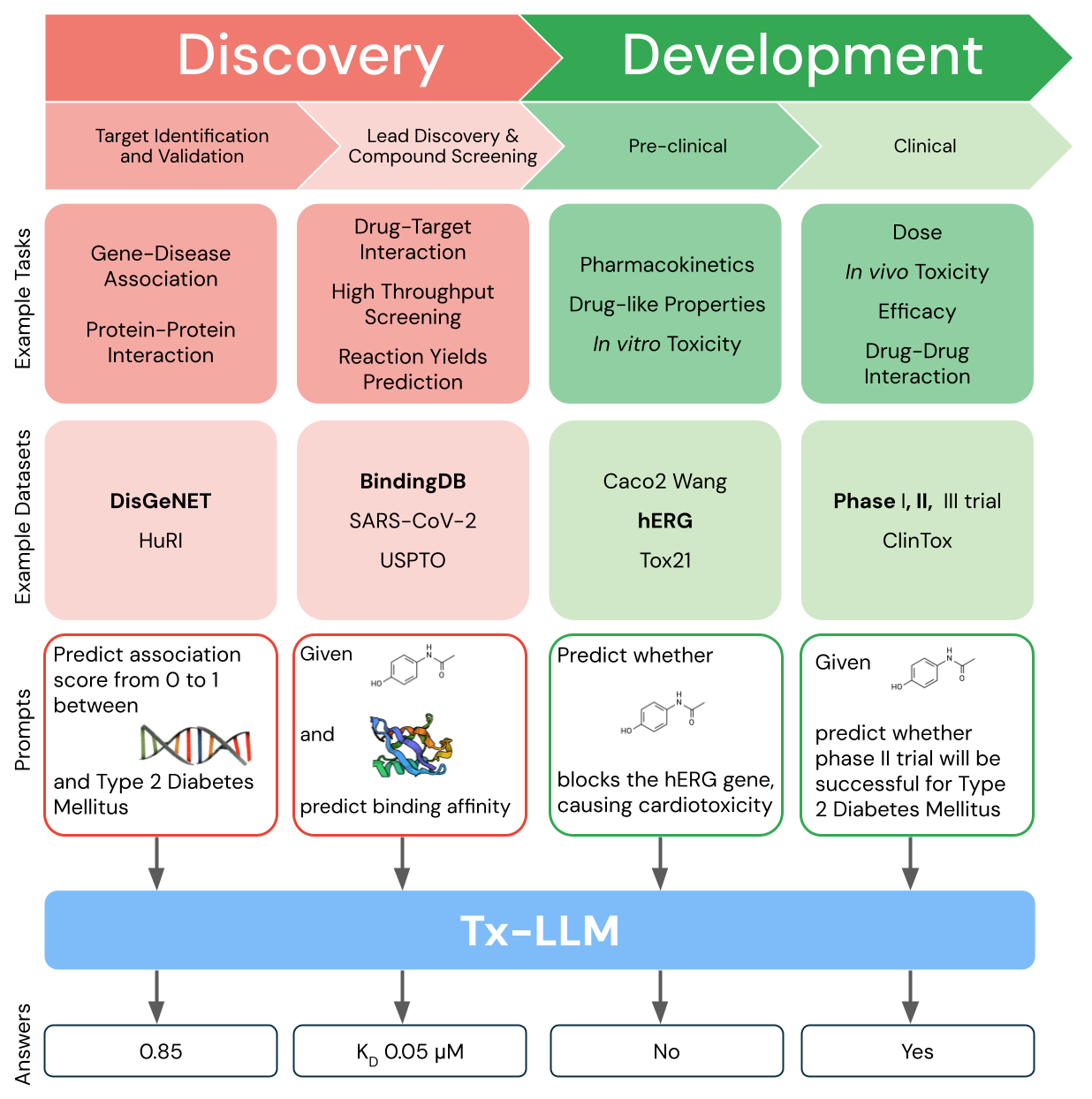
Tx-LLM is a single model that is fine-tuned to predict properties for tasks related to therapeutic development, ranging from early-stage target identification to late-stage clinical trial approval. (Credit: Google Research Blog)
Training and Methodology
The model’s development involved curating the Therapeutics Instruction Tuning (TxT) dataset, which consolidates information from the Therapeutic Data Commons (TDC) and additional literature. The TxT dataset consists of 709 datasets restructured into instruction-answer formats to facilitate effective language model training. Each prompt includes instructions, context, and few-shot exemplars to enable in-context learning. In addition, certain biological features, such as cell lines, are represented as textual information rather than mathematical objects, making better use of the model’s pre-existing natural language understanding.
Performance Evaluation
Tx-LLM was evaluated on several criteria and demonstrated competitive results. The model performed effectively on tasks that required the integration of molecular and textual data, such as predicting clinical trial outcomes based on a drug’s characteristics and the disease it targets. It also exhibited strong numerical prediction capabilities, which have traditionally been a challenge for LLMs. Overall, Tx-LLM achieved competitive results on 43 out of 66 tasks and outperformed existing models on 22 tasks, particularly those involving small molecules and text.
Limitations and Future Directions
While Tx-LLM provides a unified solution for multiple therapeutic research tasks, it still has limitations in comparison to some specialist models in specific areas. Additionally, it currently lacks the capability to provide natural language explanations for its predictions, which limits its utility for users seeking interpretability. The research team is exploring ways to improve these aspects, including integrating the Gemini family of models and expanding the model’s instruction-following abilities.
Next Steps and Collaboration Opportunities
Google Research and DeepMind are evaluating how to make Tx-LLM’s capabilities available to external researchers and are interested in collaborating with the broader research community. The research team encourages interested parties to share their use cases and explore potential collaboration opportunities. Such engagement will inform future developments and ensure that Tx-LLM’s capabilities can be maximally leveraged for therapeutic research.
For more information and potential collaboration, please refer to the Google Research Blog or the Expression of Interest form linked in the announcement.
Topics: AI & Digital