Evo 2: Largest Foundation Model for Genomic Research Across All Domains of Life
Evo 2, the largest AI foundation model for biology to date, is now publicly available via NVIDIA BioNeMo (Arc Institute's preprint), offering researchers a tool for analyzing and generating genomic sequences at an unprecedented scale. The model is a result of a collaboration between the Arc Institute, NVIDIA, Stanford University, UC Berkeley, and UC San Francisco.
Training and Model Architecture
Evo 2 was trained on 9.3 trillion DNA base pairs, spanning over 128,000 genomes from across the three domains of life—Eukarya, Prokarya, and Archaea. Compared to its predecessor, Evo 1, which focused mainly on prokaryotic genomes, Evo 2 draws from a broader and more diverse dataset, allowing it to capture a wider range of biological complexity.
The model was trained at scales of 7 billion and 40 billion parameters and can learn directly from DNA sequences to predict the functional effects of genetic variation.It also identifies a range of biological features, such as exon–intron boundaries, transcription factor binding sites, protein structural elements, and prophage genomic regions, without requiring task-specific fine-tuning.
In addition to its predictive abilities, Evo 2 can generate entire genomic sequences with improved coherence across mitochondrial, prokaryotic, and eukaryotic genomes.
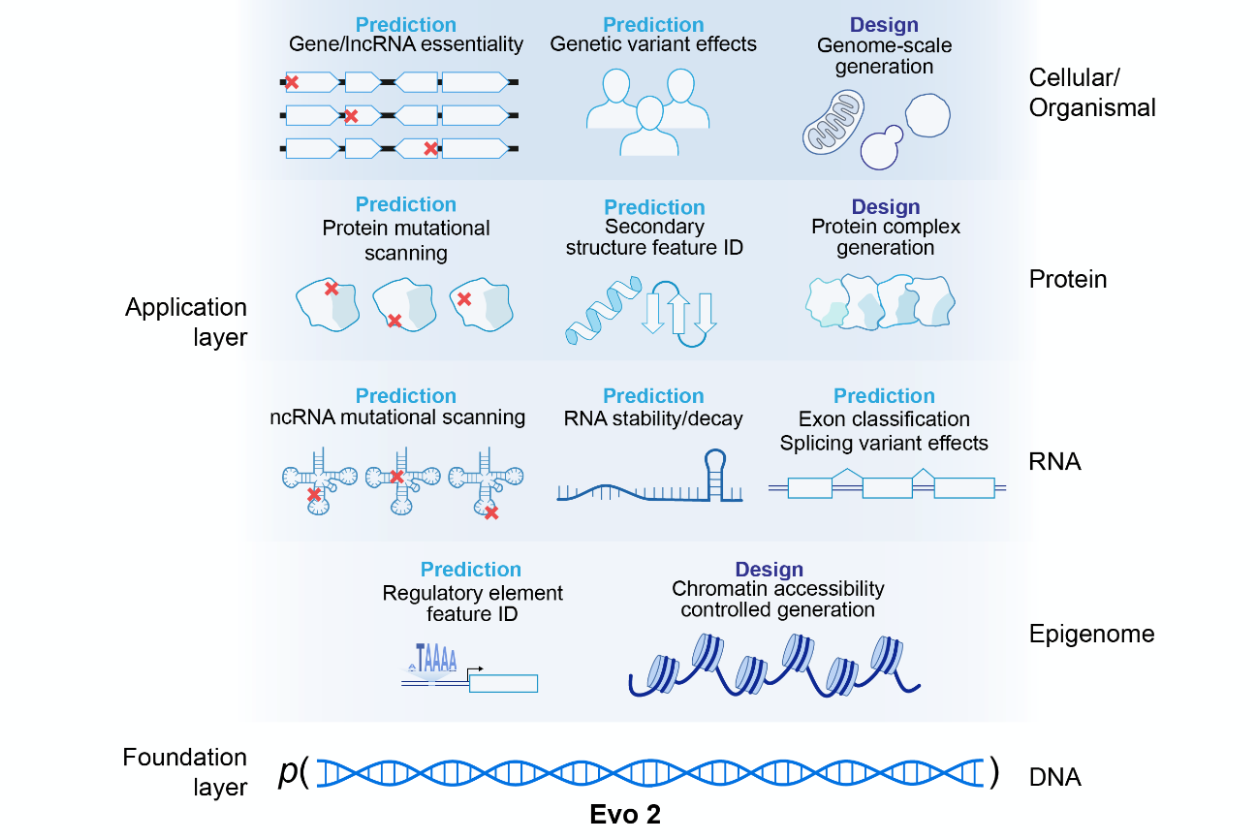
Overview of the Evo 2 model's architecture, training process, datasets, and evaluations... "Evo 2 models DNA sequences, enabling applications across the central dogma at molecular and cellular scales." — Arc Institute
The model is built on StripedHyena 2, a deep learning architecture optimized for processing long genomic sequences, with contributions from Greg Brockman, Co-Founder and President of OpenAI. StripedHyena 2 combines convolutional filters and gates to model biological sequences more efficiently than traditional Transformer-based architectures, enabling work at larger scales.
Evo 2 can process sequences up to 1 million nucleotides—about eight times longer than Evo 1—enabling the study of distant genetic interactions. Its long-context capabilities were demonstrated through a 'needle-in-a-haystack' task, successfully retrieving a 100-base-pair sequence from a 1-million-base-pair genomic segment.
Training required extensive computational resources, with NVIDIA providing access to 2,048 H100 GPUs via its DGX Cloud on AWS. This cloud-based infrastructure allowed researchers to train the model with 30 times more data than Evo 1 while improving efficiency in handling long-range dependencies within genetic sequences.
Applications in Genomics and Molecular Biology
Evo 2 is capable of generating entire genomes, including mitochondrial sequences and prokaryotic genomes. It successfully generated human mitochondrial DNA with the correct number of coding sequences (CDS), tRNA, and rRNA genes while preserving the natural order and arrangement of these genes. Additionally, the model was used to generate synthetic Mycoplasma genitalium genomes, demonstrating its potential in synthetic biology and bioengineering.
The model is designed to accelerate genomic research across multiple domains, including healthcare, synthetic biology, and environmental science. By modeling the relationships between DNA, RNA, and proteins, Evo 2 can help researchers predict how genetic variations influence biological functions and diseases.
- Variant Impact Prediction: In validation tests, Evo 2 demonstrated over 90% accuracy in predicting the functional consequences of mutations in BRCA1, a gene associated with breast cancer. The model can analyze previously uncharacterized genetic variants, helping researchers assess their potential impact on disease development.
- Genome Design: Evo 2 can generate complete genome sequences, including designing artificial genomes at the scale of simple bacteria. This capability has implications for synthetic biology, enabling researchers to engineer new biological systems with specific traits.
- Protein Engineering: By analyzing genetic sequences, Evo 2 can help design novel proteins with targeted functions, supporting applications in drug discovery and industrial biotechnology.
- Agricultural Biotechnology: Being able to analyze genomic sequences across diverse species, Evo 2 could provide insights to support research in agricultural biotechnology.
Deployment and Accessibility
Evo 2 is integrated into NVIDIA BioNeMo, where it is available as an NVIDIA NIM microservice. This allows researchers to generate biological sequences with configurable parameters, including tokenization, sampling, and temperature settings. Users can fine-tune Evo 2 on their own datasets via the open-source NVIDIA BioNeMo Framework, which provides tools for adapting pretrained models to specialized research applications.
To further support research and development, Arc Institute and its collaborators have released Evo 2’s training data, model weights, and inference code under an open-source framework. The Evo 2 codebase is publicly accessible on Arc’s GitHub, and researchers can explore its outputs through Evo Designer, a user-friendly interface for genomic sequence generation, and Evo Mech Interp Visualizer (collaboration with Goodfire), a tool for analyzing the model’s learned features (for more resources, see p.39, '6. Code and model availability').
Scientific and Ethical Considerations
Evo 2’s capabilities raise considerations regarding biosecurity and ethical AI deployment. To mitigate risks, the model’s training data excludes human pathogens, and safeguards have been implemented to prevent misuse in designing harmful biological sequences. The project also includes contributions from researchers specializing in responsible AI development, ensuring that Evo 2 aligns with best practices in ethical genomics research.
Topics: AI & Digital