Basetwo Raises $11.5M for AI-Driven, First-Principles Pharmaceutical Manufacturing Optimization
Basetwo, an AI platform for manufacturing engineers, has raised $11.5 million in Series A funding. The funding will support the development of Basetwo’s Physics AI platform, designed to optimize pharmaceutical and chemical manufacturing processes.
Scaling production from lab to commercial levels and improving efficiency remain key challenges in manufacturing. Traditional machine learning approaches, which rely on historical data, often fail to capture the underlying physics and chemistry of these processes.
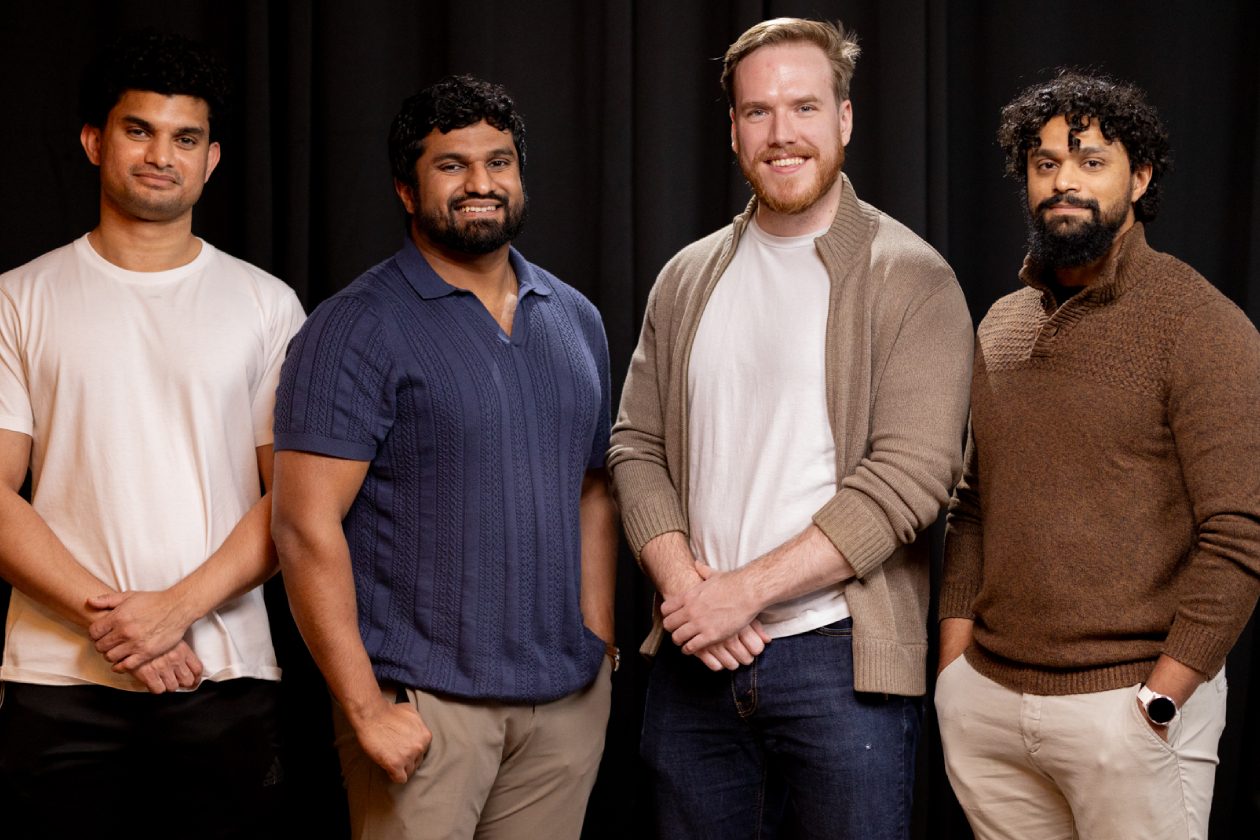
Basetwo Founders (left to right): Tawfeeq, Thouheed, Kiefer, and Thamjeeth
Basetwo’s platform is designed to combine first-principles models, based on chemical and physical engineering, with machine learning to develop hybrid models intended to simulate manufacturing processes more accurately. These simulations aim to help engineers predict system behavior under new conditions, identify inefficiencies, and test adjustments in a virtual environment before real-world implementation. By integrating these capabilities, the platform seeks to reduce reliance on costly trial-and-error methods, accelerate production cycles, and enhance product consistency and quality.
How the Basetwo Platform Works:
-
Data Integration and Preparation:
Basetwo supports integration with both offline and online data sources, allowing engineers to build robust data pipelines using a drag-and-drop interface. The platform also includes tools for cleaning, gap-filling, and transforming data, enabling seamless preparation for analysis. -
Modeling and Simulation:
The platform offers a library of industry-specific process models that engineers can customize using its drag-and-drop editor. Any gaps in process knowledge are addressed with machine learning models, and hybrid modeling capabilities allow the combination of physics-based principles with machine learning to improve predictions. -
Visualization and Optimization:
Engineers can overlap time-series datasets to identify correlations and visualize trends, while advanced statistical tools such as principal component analysis reveal key variables contributing to process outcomes. Users can conduct virtual "what-if" experiments to test process adjustments and determine optimal production setpoints under specific constraints. -
Operationalization and Deployment:
Once optimized, outcomes can be integrated into existing enterprise dashboards or operationalized across teams with automated notifications and workflow integrations, such as Office 365. The platform also supports exporting models to external simulation tools for extended use.
Reportedly, customers using the platform have reported notable improvements, including up to 40% faster cycle times, 25% higher product quality, and reduced raw material waste.
Topics: Manufacturing & Pharma 4.0