Swiss Researchers Advance Spatial Proteomics with AI-Powered Virtual Tissue Foundation Model
Researchers from École Polytechnique Fédérale de Lausanne (EPFL) and ETH Zurich, in collaboration with the University Hospital Zurich and the University of Zurich, have introduced VirTues (Virtual Tissues), a foundation model framework for analyzing tissue architecture using spatial proteomics data.
This work builds on the framework laid out in "How to Build the Virtual Cell with Artificial Intelligence: Priorities and Opportunities" (Cell, 2024), which outlined a vision for creating multi-scale, multi-modal AI models capable of representing biological systems across molecular, cellular, and tissue levels. The perspective identified major challenges in modeling cellular processes, including the complexity of multi-scale interactions, the diversity of cellular functions, and the nonlinear dynamics of biological systems. Traditional rule-based models often fail to adequately simulate such processes, emphasizing the need for data-driven approaches.
The proposed AI virtual cell (AIVC) framework in the earlier work aimed to overcome these challenges by integrating advances in AI and omics. It envisioned a foundational framework that combines biological data across modalities, scales, and contexts to produce universal representations of cells and tissues. These representations would be predictive, generative, and queryable, offering tools to simulate, explore, and predict cellular behaviors under various conditions, such as disease states or experimental perturbations.
VirTues directly applies these principles to tackle the computational challenges of multiplex tissue imaging, a method that captures spatially organized molecular data across dozens of protein and RNA markers. The model is designed to analyze such high-dimensional data while accommodating variability in marker combinations across datasets. Its vision transformer architecture integrates spatial and molecular dimensions, enabling representations that span molecular, cellular, and tissue scales. This approach reflects the hierarchical modeling outlined in the earlier vision paper, emphasizing the integration of molecular information to better understand tissue organization and function.
A notable feature of VirTues is its ability to generalize to new datasets and diseases without retraining. Trained on imaging data from cancers such as lung, breast, and melanoma, the model demonstrates strong zero-shot capabilities, performing well on previously unseen diseases like pancreatic tissue in type 1 diabetes. This capability aligns with the objective of building adaptable models capable of interpreting diverse biological systems and experimental contexts.
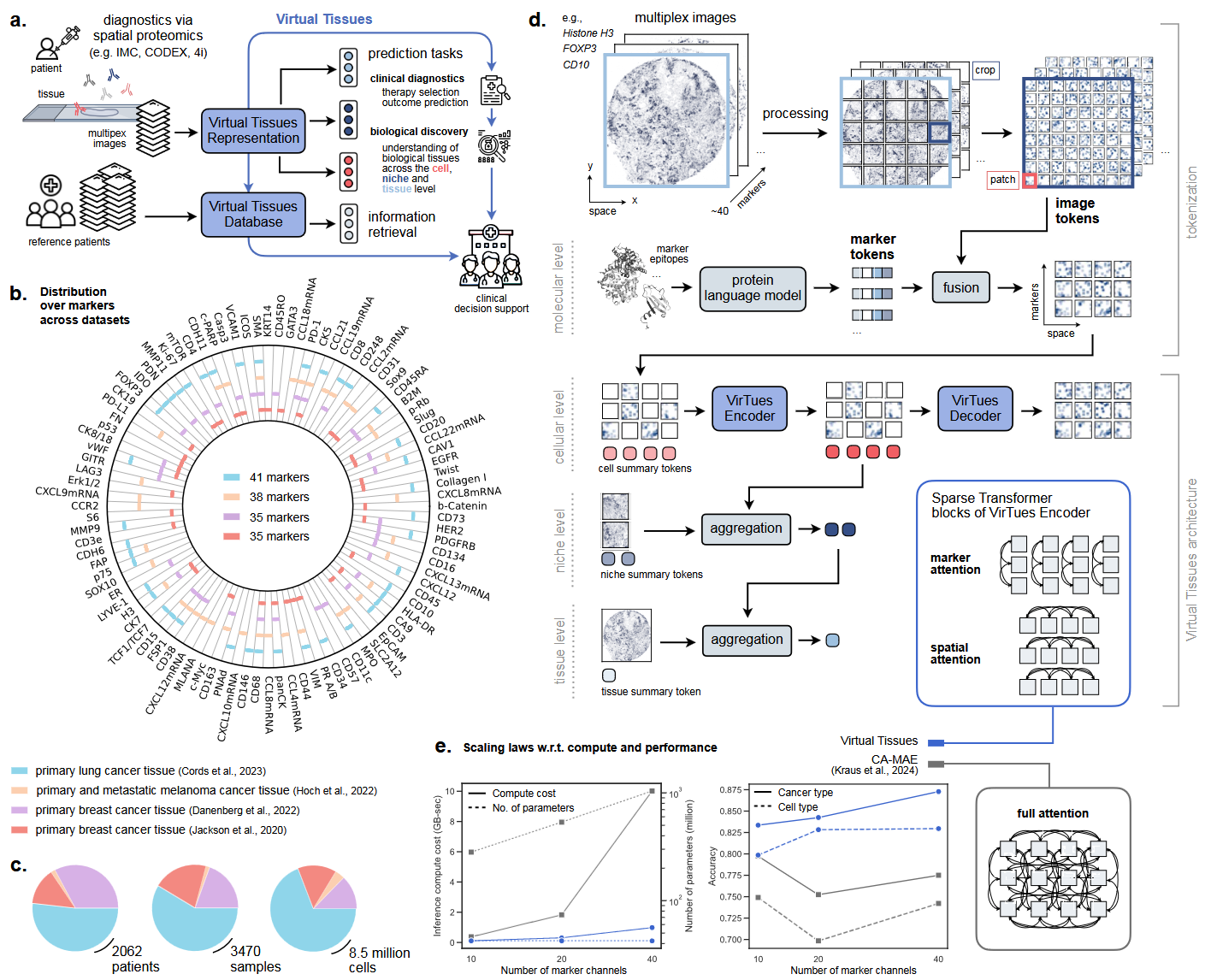
Overview of the Virtual Tissues platform. (a) VirTues maps multiplexed tissue images to virtual tissue representations for clinical and biological predictions across cell, niche, and tissue levels, with a database enabling retrieval of similar samples for clinical decision support. (b) It is trained on four IMC datasets from lung, breast, and melanoma cancers, covering 96 protein and mRNA markers. (c) Dataset sizes include patients, biopsy samples, and cells. (d) Multiplexed images are processed into 3D grids of image tokens, with marker tokens fused to image tokens using a protein language model, enabling hierarchical tissue representation. (e) Computational cost and performance are compared between VirTues and CA-MAE16 based on the number of utilized markers. (Source: arXiv:2501.06039v1 [q-bio.QM] 10 Jan 2025)
VirTues supports practical applications across research and clinical tasks. It reconstructs masked regions of tissue imaging data, predicts features such as cancer grade and likelihood of relapse, and retrieves comparable patient cases by mapping tissue architectures in a Virtual Tissues Database. These applications are made possible by VirTues’ multi-scale approach, which captures relationships between markers and spatial tissue organization. The model's attention mechanisms highlight specific markers and spatial regions within tissues that are most relevant to its predictions. This functionality allows researchers to trace how molecular and spatial features contribute to its outputs, supporting detailed analyses for clinical diagnostics and biological investigations.
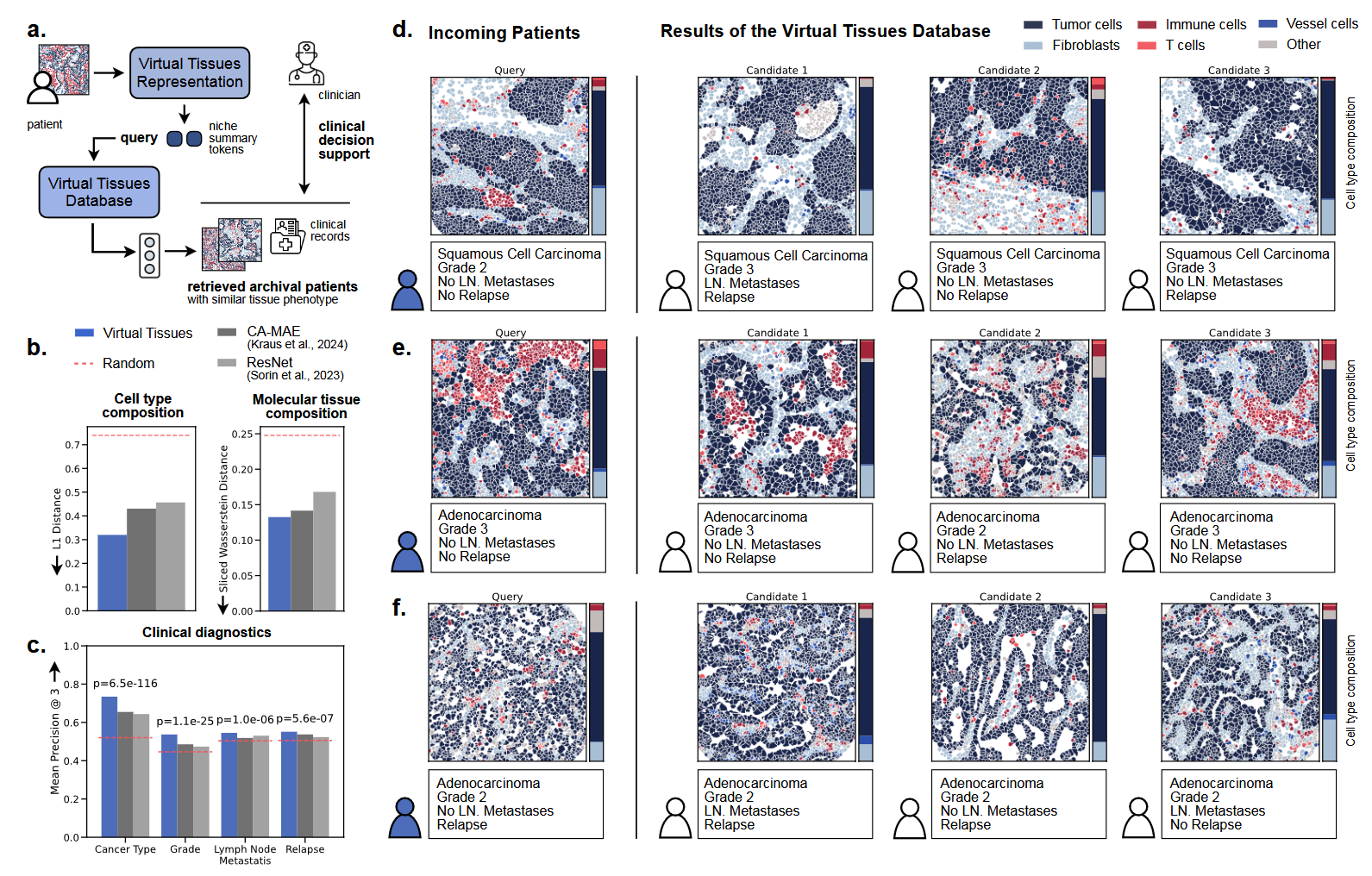
Clinical decision support through Virtual Tissues Database. (a) VirTues enables data-driven clinical decision support by retrieving similar patient cases using niche summary tokens and an optimal transport-based retrieval system. (b) Bar plots compare average similarity scores for cell type composition (L1 distance) and molecular composition (sliced Wasserstein distance) between query tissues and closest matches, evaluated against random retrieval. (c) Mean precision of the top-3 retrieval results is shown for four clinical labels: cancer type, grade, lymph node metastasis, and relapse, with P-values from McNemar tests indicating statistical significance. (d-f) Exemplary retrieval results display query tissues alongside their three closest matches, with tissues represented by color-coded cell type masks and corresponding proportional cell type compositions. (Source: arXiv:2501.06039v1 [q-bio.QM] 10 Jan 2025)
VirTues’ Virtual Tissues Database further extends its utility by enabling data-driven clinical decision support. The database organizes tissue representations into a structured system, allowing retrieval of similar patient cases based on molecular profiles, tissue architecture, and clinical outcomes. This retrieval system uses niche-level embeddings and optimal transport-based similarity metrics to identify comparable cases across patient cohorts. For example, clinicians could use the database to find cases with analogous tissue phenotypes and review their associated treatments and outcomes, aiding in evidence-based decision-making.
The model’s ability to handle diverse datasets and marker combinations also facilitates cross-study comparisons, enabling meta-analyses and the integration of new data types without retraining. By creating a unified framework for analyzing tissue imaging data, VirTues aims to bridge the gap between computational and experimental approaches, supporting both exploratory research and practical applications in precision medicine.
This work shows the potential feasibility of applying foundational AI models to analyze complex biological data at multiple scales, a concept central to the earlier vision for AI virtual cells. VirTues provides a computational framework that integrates molecular and spatial data, offering tools for better understanding tissue architecture and its role in disease.
Notably, the concept of a virtual cell is gaining traction across the biotechnology and AI communities, with initiatives like Noetik’s OCTO-VirtualCell and Recursion’s post-merger development of a world model platform among others. Some other efforts include the Chan Zuckerberg Initiative’s integration of datasets like the Human Cell Atlas for cellular modeling and Google DeepMind’s exploration of virtual cells to simulate molecular dynamics and aid in drug discovery.
Topics: AI & Digital