Profluent Unveils AI Model to Advance CRISPR-Cas Systems for Gene Editing
Profluent Bio, a Berkeley-based biotech founded in 2022, and funded with $44 million to date, has released an AI model aimed at advancing the design of CRISPR-Cas gene-editing systems. The company's latest research, published in a preprint on January 6th, 2025, demonstrates how deep learning can be harnessed to expand the genome-editing potential of CRISPR complexes by tailoring their DNA recognition capabilities.
At the core of Profluent’s innovation is Protein2PAM, a deep learning model trained on over 45,000 evolutionary CRISPR-Cas datasets. The model is designed to predict and customize the protospacer-adjacent motif (PAM) recognition of CRISPR enzymes, a key factor in determining which genomic sites these systems can edit. The study highlights Protein2PAM’s ability to computationally evolve Cas enzymes.
By enabling the customization of Cas proteins for specific PAMs, Profluent’s approach could overcome one of the major constraints of existing CRISPR systems: their limited targeting range. According to the study, Protein2PAM successfully engineered Nme1Cas9 variants capable of editing previously inaccessible genomic regions, expanding the utility of CRISPR technologies for therapeutic and research applications.
AI Meets Protein Design
Profluent’s foundation models utilize AI to design proteins inspired by natural scaffolds or created from scratch. By integrating computational tools with in-house wet lab capabilities, the company aligns protein design steps with specific practical applications, enabling precision and scalability in addressing biological challenges.
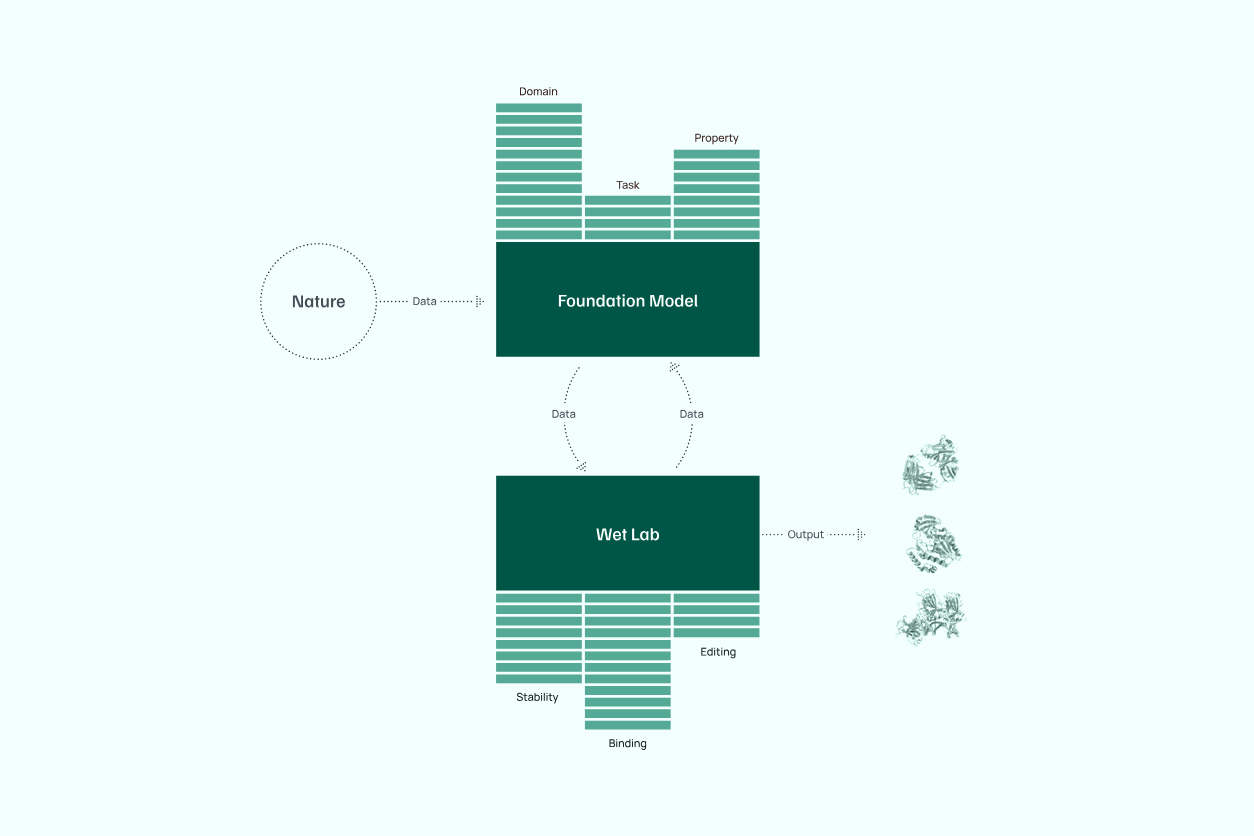
From Profluent's technical overview
In August 2024, Profluent also introduced ProseLM (protein structure-encoded language model), a tool that incorporates structural and functional context into protein language models for targeted protein design. ProseLM integrates atomic-level details—such as interactions with nucleic acids, ligands, and ions—using adapter layers added to pre-trained language models. This approach makes ProseLM computationally efficient while enabling precise, context-specific sequence generation.
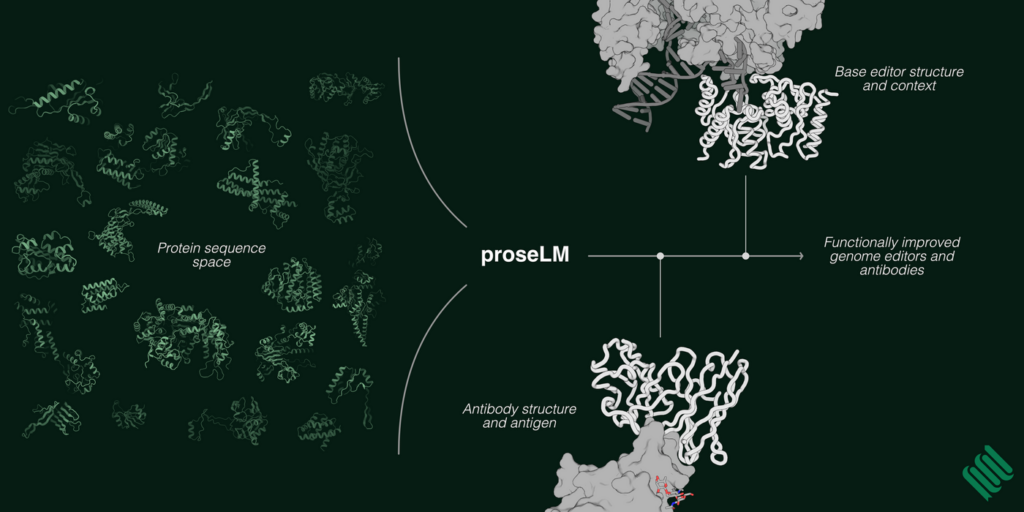
Credit: Profluent Bio
In experimental validation, ProseLM demonstrated nearly 50% higher efficiency in base editing compared to starting designs and improved antibody binding affinity by up to 2.5 times for therapeutic antibodies like nivolumab. The model builds on Profluent’s earlier success with OpenCRISPR-1, a programmable gene editor released publicly in April 2024 for research and commercial applications.
Topics: Tools & Methods